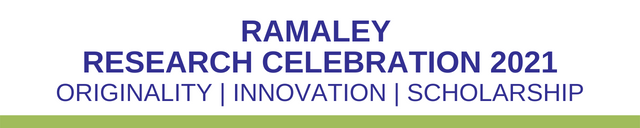
Presentation Title
Automatic Polyp Detection in Gastroscopy Using Deep Learning
Abstract
Automatic Polyp Detection in Gastroscopy Using Deep Learning
Himanshu Bhushan, Ming Ma
Department of Computer Science, Winona State University, 225 W King St, Winona, MN 55987, USA
Polyp detection in gastroscopy has attracted much researchers’ attention over the past few decades. Early detection and removal of polyps plays a crucial role in gastric cancer prevention. However, it is a challenging task for physicians to detect various types of polyps. To facilitate this process, we propose to use a deep learning based method for automatic polyp detection. The proposed method employs the YOLO model that is based on the convolutional neural network (CNN). The model is trained and tested in a dataset of 758 images obtained during gastroscopy. Out of these 758 images, 606 images were used for training and 152 were used for testing. Experimental results show that the proposed method achieved 85%-95% image precision on the testing dataset. With the Intersection Over Union (IoU) being 0.5, the mean area precision (mAP) ranged from 81%-92%. The trained model can detect the polyps on 10 testing images in 0.226 seconds. This demonstrates that the proposed method can help physicians efficiently detect the gastric polyp and has the potential to be applicable in clinical practice.
College
College of Science & Engineering
Department
Computer Science
Location
Winona, Minnesota
Presentation Type
Video (Prerecorded-MP4)
Automatic Polyp Detection in Gastroscopy Using Deep Learning
Winona, Minnesota
Automatic Polyp Detection in Gastroscopy Using Deep Learning
Himanshu Bhushan, Ming Ma
Department of Computer Science, Winona State University, 225 W King St, Winona, MN 55987, USA
Polyp detection in gastroscopy has attracted much researchers’ attention over the past few decades. Early detection and removal of polyps plays a crucial role in gastric cancer prevention. However, it is a challenging task for physicians to detect various types of polyps. To facilitate this process, we propose to use a deep learning based method for automatic polyp detection. The proposed method employs the YOLO model that is based on the convolutional neural network (CNN). The model is trained and tested in a dataset of 758 images obtained during gastroscopy. Out of these 758 images, 606 images were used for training and 152 were used for testing. Experimental results show that the proposed method achieved 85%-95% image precision on the testing dataset. With the Intersection Over Union (IoU) being 0.5, the mean area precision (mAP) ranged from 81%-92%. The trained model can detect the polyps on 10 testing images in 0.226 seconds. This demonstrates that the proposed method can help physicians efficiently detect the gastric polyp and has the potential to be applicable in clinical practice.
Comments
We used a deep learning based method for automatic polyp detection.