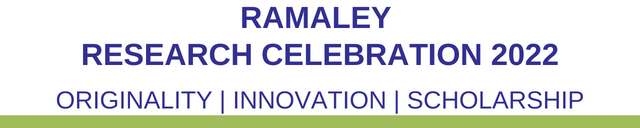
Presentation Title
CT Image Segmentation for Prostate Cancer Diagnosis Based on 3D U-net Deep Learning Model
Abstract
The vast advancement in deep learning techniques over the past years has consequently led to the creation of state-of-the-art models applied in the medical imaging field for cancer diagnosis. The basic 3D U-net model has shown success in delimiting various organs on medical images such as CT scans, MRIs, and X-Rays. However, it is desirable to develop a more accurate segmentation model. In this work, we study the basic 3D U-net model applied to CT image segmentation of the male prostate organs for prostate cancer diagnosis and compare its performance with that of an improved 3D U-net model. Experiments are carried out on a dataset of CT images with prostate organs and the performance is evaluated using the Intersection over Union (IoU) metric.
College
College of Science & Engineering
Department
Computer Science
Location
Kryzsko Commons Ballroom, Winona, Minnesota
Start Date
4-20-2022 10:00 AM
End Date
4-20-2022 11:00 AM
Presentation Type
Poster Presentation
Session
1b=10am-11am
Poster Number
38
CT Image Segmentation for Prostate Cancer Diagnosis Based on 3D U-net Deep Learning Model
Kryzsko Commons Ballroom, Winona, Minnesota
The vast advancement in deep learning techniques over the past years has consequently led to the creation of state-of-the-art models applied in the medical imaging field for cancer diagnosis. The basic 3D U-net model has shown success in delimiting various organs on medical images such as CT scans, MRIs, and X-Rays. However, it is desirable to develop a more accurate segmentation model. In this work, we study the basic 3D U-net model applied to CT image segmentation of the male prostate organs for prostate cancer diagnosis and compare its performance with that of an improved 3D U-net model. Experiments are carried out on a dataset of CT images with prostate organs and the performance is evaluated using the Intersection over Union (IoU) metric.