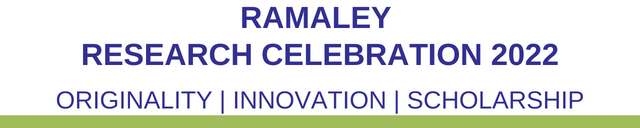
Presentation Title
Abstract
Action recognition in various sports is becoming increasingly more popular mostly due to its uses for analysis and training by coaches. Using action recognition specifically for tennis can be a very helpful tool to put together a collection of different precise strokes and actions that can be analyzed by coaches for training. However, it is a challenging task to design an accurate action recognition model which can provide feedback for a stroke or action preformed in a tennis match. Some existing method uses a deep learning model pretrained on ImageNet and trains it on a single LSTM (Long Short-Term Memory) layer. In this work, we propose to use two or more LSTM layers to help improve the model. Experiments are conducted on a dataset of 1980 RGB videos. The results in the evaluation experiments show that the improved model achieves an increase in the f1-score.
College
College of Science & Engineering
Department
Computer Science
Location
Kryzsko Commons Ballroom, Winona, Minnesota
Start Date
4-20-2022 1:00 PM
End Date
4-20-2022 2:00 PM
Presentation Type
Poster Presentation
Session
2a=1pm-2pm
Poster Number
17
Included in
Tennis Action Recognition Based on a Deep Learning Model.
Kryzsko Commons Ballroom, Winona, Minnesota
Action recognition in various sports is becoming increasingly more popular mostly due to its uses for analysis and training by coaches. Using action recognition specifically for tennis can be a very helpful tool to put together a collection of different precise strokes and actions that can be analyzed by coaches for training. However, it is a challenging task to design an accurate action recognition model which can provide feedback for a stroke or action preformed in a tennis match. Some existing method uses a deep learning model pretrained on ImageNet and trains it on a single LSTM (Long Short-Term Memory) layer. In this work, we propose to use two or more LSTM layers to help improve the model. Experiments are conducted on a dataset of 1980 RGB videos. The results in the evaluation experiments show that the improved model achieves an increase in the f1-score.