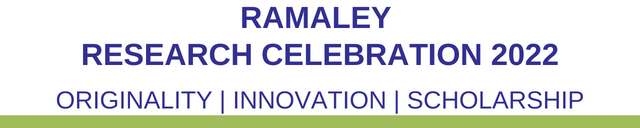
Presentation Title
Comparing Geospatial Interpolation Methods for Modeling Snow Depths
Abstract
Predicting values of unknown points at geographic locations using known points at other locations seems simple, but many methods offer different solutions to try to predict such points. The goal of this research was to investigate how different geospatial interpolation methods work by comparing their accuracy of predicting snow depths in western Colorado. This data is publicly available and was collected by the Remote Sensing Center at the University of Alabama, who used an FMCW radar system to measure the snow depths. The interpolation methods explored in this project included ordinary kriging, inverse distance weighting, k nearest neighbor, thin plate splines, and universal kriging. Out of all these methods, inverse distance weighting performed the best on this subset of snow depths data.
College
College of Science & Engineering
Department
Mathematics & Statistics
Location
Kryzsko Commons Ballroom
Start Date
4-20-2022 2:00 PM
End Date
4-20-2022 3:00 PM
Presentation Type
Poster Presentation
Session
2b=2pm-3pm
Poster Number
8
Comparing Geospatial Interpolation Methods for Modeling Snow Depths
Kryzsko Commons Ballroom
Predicting values of unknown points at geographic locations using known points at other locations seems simple, but many methods offer different solutions to try to predict such points. The goal of this research was to investigate how different geospatial interpolation methods work by comparing their accuracy of predicting snow depths in western Colorado. This data is publicly available and was collected by the Remote Sensing Center at the University of Alabama, who used an FMCW radar system to measure the snow depths. The interpolation methods explored in this project included ordinary kriging, inverse distance weighting, k nearest neighbor, thin plate splines, and universal kriging. Out of all these methods, inverse distance weighting performed the best on this subset of snow depths data.