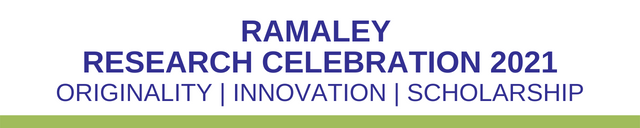
Presentation Title
Using Support Vector Machine Learning to Predict Game Entity in a “Super Smash Bros. Melee” game
Abstract
Over the years fighting games have gotten more refined as in-game character mechanics have grown significantly more complex. With software advancements, players of the popular platform fighting game Super Smash Bros. Melee (SSBM) are able to save replay files of matches and extract metadata from previously played matches. Information from matches can be used to train classification models to predict aspects of the game such as the character played. With a diverse cast of characters to choose from, each character has a unique move set to use during a match. However with the complex nature of the SSBM in-game environment on top of the multi-player element of the game, the ways in which a character can be controlled is nearly limitless. With a refined metagame of many characters, but the dynamic ability of the game environment, this poses as a clash between the predictability of certain characters and the mind games of the human player. Nonetheless, this project aims to show the predictability in different characters within a SSBM match, using information and metadata extracted from previous replay files of matches to train a Support Vector Machine learning model.
College
College of Science & Engineering
Department
Computer Science
Location
Winona, Minnesota
Breakout Room
10
Start Date
4-14-2021 1:00 PM
End Date
4-14-2021 1:45 PM
Presentation Type
Video (Live-Zoom)
Using Support Vector Machine Learning to Predict Game Entity in a “Super Smash Bros. Melee” game
Winona, Minnesota
Over the years fighting games have gotten more refined as in-game character mechanics have grown significantly more complex. With software advancements, players of the popular platform fighting game Super Smash Bros. Melee (SSBM) are able to save replay files of matches and extract metadata from previously played matches. Information from matches can be used to train classification models to predict aspects of the game such as the character played. With a diverse cast of characters to choose from, each character has a unique move set to use during a match. However with the complex nature of the SSBM in-game environment on top of the multi-player element of the game, the ways in which a character can be controlled is nearly limitless. With a refined metagame of many characters, but the dynamic ability of the game environment, this poses as a clash between the predictability of certain characters and the mind games of the human player. Nonetheless, this project aims to show the predictability in different characters within a SSBM match, using information and metadata extracted from previous replay files of matches to train a Support Vector Machine learning model.